MultiSciView: Multivariate Scientific X-ray Image Visual Exploration with Cross-Data Space Views
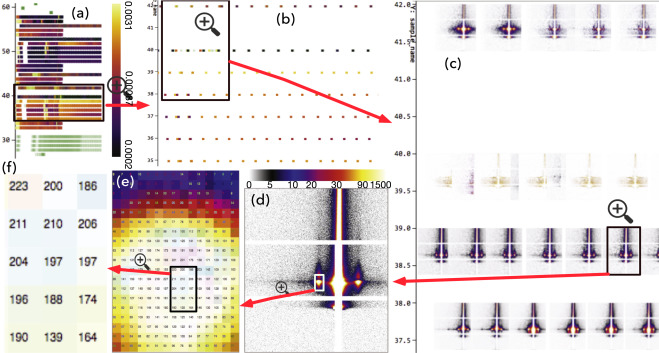
Citation
Zhong, W.; Xu, W.; Yager, K.G.; Doerk, G.S.; Zhao, J.; Tian, Y.; Ha, S.; Xie, C.; Zhong, Y.; Mueller, K.; Kleese van Dam, K. "MultiSciView: Multivariate Scientific X-ray Image Visual Exploration with Cross-Data Space Views"
Visual Informatics 2018,
2 14–25.
doi: 10.1016/j.visinf.2018.04.003Summary
We demo a zooming user interface (ZUI) that allows one to explore large x-ray scattering datasets. Tools are provided for organizing and re-organize data to look for trends, and to zoom between scatterplots of trends, through neighbourhoods of scattering images, down to individual pixel values in detector images.
Abstract
X-ray images obtained from synchrotron beamlines are large-scale, high-resolution and high-dynamic-range grayscale data encoding multiple complex properties of the measured materials. They are typically associated with a variety of metadata which increases their inherent complexity. There is a wealth of information embedded in these data but so far scientists lack modern exploration tools to unlock these hidden treasures. To bridge this gap, we propose MultiSciView, a multivariate scientific x-ray image visualization and exploration system for beamline-generated x-ray scattering data. Our system is composed of three complementary and coordinated interactive visualizations to enable a coordinated exploration across the images and their associated attribute and feature spaces. The first visualization features a multi-level scatterplot visualization dedicated for image exploration in attribute, image, and pixel scales. The second visualization is a histogram-based attribute cross filter by which users can extract desired subset patterns from data. The third one is an attribute projection visualization designed for capturing global attribute correlations. We demonstrate our framework by ways of a case study involving a real-world material scattering dataset. We show that our system can efficiently explore large-scale x-ray images, accurately identify preferred image patterns, anomalous images and erroneous experimental settings, and effectively advance the comprehension of material nanostructure properties.