Automatic X-ray Scattering Image Annotation via Double-View Fourier-Bessel Convolutional Networks
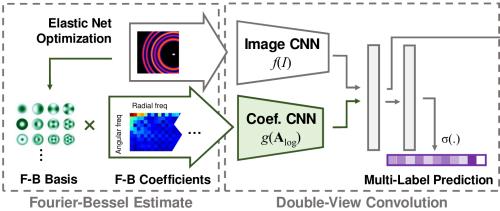
Citation
Guan, Z.; Qin, H.; Yager, K.G.; Choo, Y.; Yu, D. "Automatic X-ray Scattering Image Annotation via Double-View Fourier-Bessel Convolutional Networks"
British Machine Vision Conference 2018,
0828 1–10.
url: http://bmvc2018.org/contents/papers/0828.pdfSummary
We use a 'physics-aware' deep learning method to automatically classify x-ray scattering images. We exploit a multi-channel architecture, where different data representations are used to improve performance, and the data transformations used as inputs to the different channels are tuned to be 'natural' for the given scientific problem. In particular, two convolutional neural networks are used to extract information from two complementary views of input x-ray scattering images. The first channel/view is the raw detector image. The second channel/view remaps the data into a matrix of Fourier-Bessel coefficients; this representation explicitly highlights symmetry information from the data. The combined system achieves record-setting performance.
Abstract
X-ray scattering is a key technique towards material analysis and discovery. Modern x-ray facilities are producing x-ray scattering images at such an unprecedented rate that machine aided intelligent analysis is required for scientific discovery. This paper articulates a novel physics-aware image feature transform, Fourier-Bessel transform (FBT), in conjunction with deep representation learning, to tackle the problem of annotating x-ray scattering images with a diverse label set of physics characteristics. We devise a novel joint inference model, Double-View Fourier-Bessel Convolutional Neural Network (DVFB-CNN) to integrate feature learning in both polar frequency and image domains. For polar frequency analysis, we develop an FBT estimation algorithm for partially observed x-ray images, and train a dedicated CNN to extract structural information from FBT. We demonstrate that our deep Fourier-Bessel features well complement standard convolutional features, and the joint network (i.e., DVFB-CNN) improves mean average precision by 13% in multilabel annotation. We also conduct transfer learning on real experimental datasets to further confirm that our joint model is well generalizable.