Non-Blind Deblurring for Fluorescence: A Deformable Latent Space Approach With Kernel Parameterization
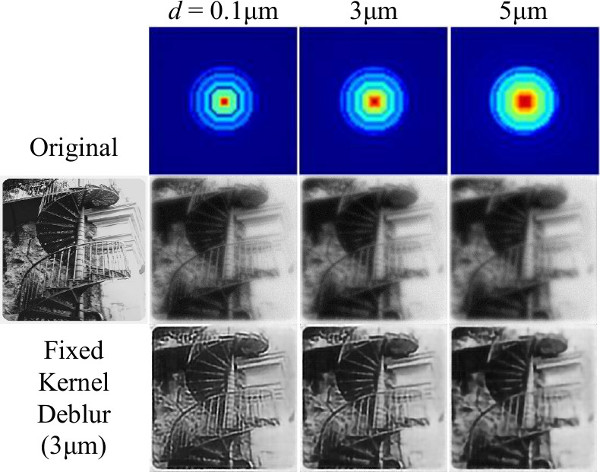
Citation
Guan, Z.; Tsai, E.H.R.; Huang, X.; Yager, K.G.; Hong, Q. "Non-Blind Deblurring for Fluorescence: A Deformable Latent Space Approach With Kernel Parameterization"
Applications of Computer Vision (WACV) 2022,
1 711–719.
Summary
We apply machine-learning neural network approaches to the problem of deblurring x-ray fluorescence imagery.
Abstract
Non-blind deblurring (NBD) is a modeling method of the image deblurring problem in computer vision, where the blurring kernel is known or can be externally estimated. In this paper, we attempt to solve a parametric NBD problem, inspired by the simultaneous acquisition of ptychography and fluorescent imaging (FI). Ptychography is an imaging method that favors larger probes, i.e. convolutional kernels, while FI relies on a small probe for high resolution. Also, the kernel can be solved during ptychographic reconstruction. With Ptycho-FI using the same larger kernel, we can perform NBD on the blurred fluorescent images to achieve high-resolution FI, and thus speed up the experiments. To this end, we design a deep latent space deformation network that is directly parameterized by the kernel. The network consists of three components: encoder, deformer, and decoder, where the deformer is specifically meant to rectify the latent space representations of blurred images to a standard latent space, regardless of the kernel. The deformation network is trained with a two-stage training scheme. We conduct extensive experiments to confirm that our parametric model can adapt to drastically different blurring kernels and perform robust deblurring.