Interactive Visual Study of Multiple Attributes Learning Model of X-Ray Scattering Images
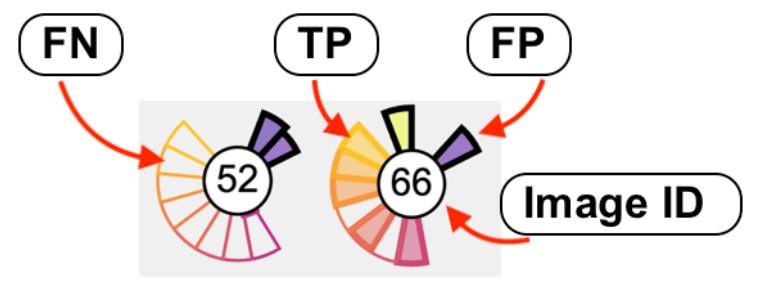
Citation
Huang, X.; Jamonnak, S.; Zhao, Y.; Wang, B.; Hoai, M.; Yager, K.G.; Xu, W. "Interactive Visual Study of Multiple Attributes Learning Model of X-Ray Scattering Images"
IEEE Transactions on Visualization and Computer Graphics 2021,
27 1312–1321.
doi: 10.1109/TVCG.2020.3030384Summary
We demonstrate a user interface tool for inspecting the performance and behavior of deep learning models.
Abstract
Existing interactive visualization tools for deep learning are mostly applied to the training, debugging, and refinement of neural network models working on natural images. However, visual analytics tools are lacking for the specific application of x-ray image classification with multiple structural attributes. In this paper, we present an interactive system for domain scientists to visually study the multiple attributes learning models applied to x-ray scattering images. It allows domain scientists to interactively explore this important type of scientific images in embedded spaces that are defined on the model prediction output, the actual labels, and the discovered feature space of neural networks. Users are allowed to flexibly select instance images, their clusters, and compare them regarding the specified visual representation of attributes. The exploration is guided by the manifestation of model performance related to mutual relationships among attributes, which often affect the learning accuracy and effectiveness. The system thus supports domain scientists to improve the training dataset and model, find questionable attributes labels, and identify outlier images or spurious data clusters. Case studies and scientists feedback demonstrate its functionalities and usefulness.