Research Insights
- Flow of Reasoning: Efficient Training of LLM Policy with Divergent Thinking. Chain-of-thought and self-critique approaches try to invoke multi-step LLM reasoning. But the process is still linear. Some newer approaches (tree-of-thought, mixture-of-agents, etc.) try to leverage parallel consideration to improve diversity. Flow-of-reasoning tries to create a tree of reasoning paths to improve diversity, using Markovian flow modeling.
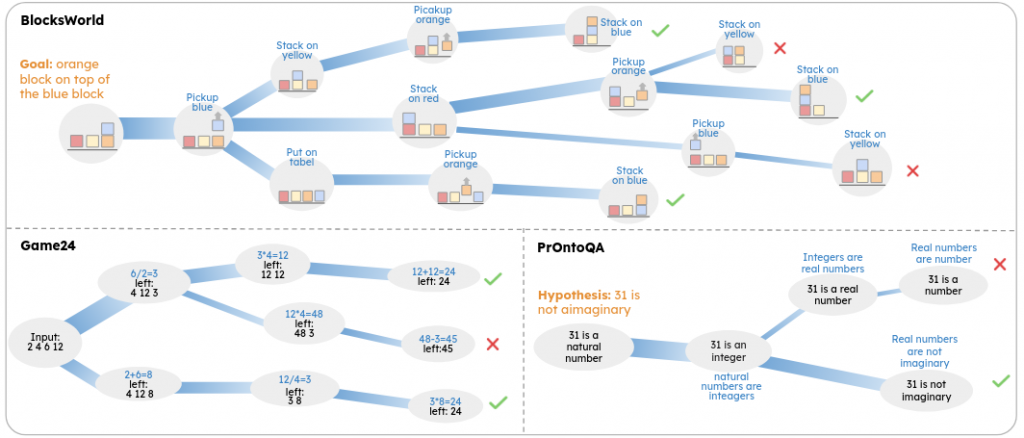
- LLMs struggle with math and logic. There are efforts to add-in or train on logic schemes (symbolic chain-of-thought, symbolic solver). New preprint: Teaching Transformers Causal Reasoning through Axiomatic Training, demonstrates training on causal axioms can work.
- Human-like Episodic Memory for Infinite Context LLMs. It is obvious that current LLMs lack the long-term memory that humans leverage to address new problems. This work tries to cluster tokens into episodes that are efficiently stored and later retrieved.
- AgentInstruct: Toward Generative Teaching with Agentic Flows. Framework generates synthetic data for training other models, that is higher-quality and more diverse than prior methods.
- Transformer Layers as Painters, analyzes how LLMs operate. They intentionally skip layers, or swap layer execution order (strong similarities to Tegmark’s “Stages of Inference” paper, c.f.). They find the LLM degrades gracefully, which suggests that every layer matters (is performing a distinct computation) but also that subsequent middle layers are operating on a common representation. They find that math-heavy tasks are most sensitive (biggest degradation). They show that middle layers can even be applied in parallel instead of sequentially (optionally looping over this parallel block). This could suggest some alternative architectures with faster inference.
AI Agents
- Decomposing Agency — capabilities without desires. Goes through different possible splits between the crucial components for a fully-featured agent (goals, awareness, planning, capabilities). An important point is that one can build different kinds of agents, with subsets of these components. E.g. the high-level motivating goals can come from the human, such that the AI agent has no goals of its own.
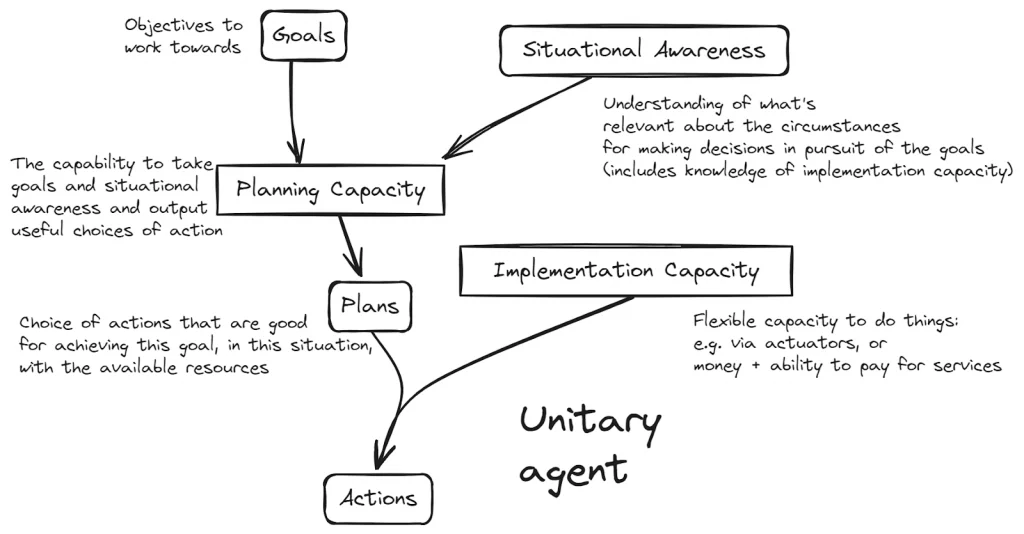
LLM
- SpreadsheetLLM: Encoding Spreadsheets for Large Language Models. They customize the encoding of a spreadsheet, allowing the LLM to reason on the semi-structured data organization of spreadsheets. Given how much data and workflow complexity can be captured by spreadsheets (combined with the availability of spreadsheet training data), this seems like a useful generic capability.
- OpenAI published some guidelines on how to improve the accuracy of LLM output.
- OpenAI posted: Prover-Verifier Games improve legibility of language model outputs. They use a strong LLM to generate answers/proofs in a way that a weaker model could verify them. This sacrifices a bit of performance, but increases legibility to humans.
- OpenAI added a “mini” version of GPT-4o to its model lineup. It is meant to be the most capable and cost-effective model.
Multi-modal Models
- Llava-NeXT-Interleave is a new group of vision-language models trained on image, video and 3D data (demo).
Chatbots
- The Pantheon Interface is a new idea for how to interact with LLMs (live instance, code). In a traditional interaction, you prompt the bot and it replies in a turn-by-turn manner. Pantheon instead invites you to type out your thoughts, and various agents will asynchronously add comments or questions to spur along your brainstorming.
- This could be an element of the human-computer interface for my proposed science exocortex (swarm of AI agents that help researchers).
- Loom is a somewhat related idea, where one have LLMs created branched writings.
Vision
- Nvidia MambaVision models use a hybrid mamba-transformer. State-of-the-art in performance and throughput. Can be applied to classification, detection, segmentation, etc.
Images
- This is a fun demo of using a physical interface to tune image synthesis model parameters, making it easier to explore the latent space.
Video
- Realtime implementations of LivePortrait are appearing (gradio app, fal.ai).
- A new text-to-video/image-to-video option: haiper.ai (examples).
World Synthesis
- A weakness of Gaussian splats are that they bake-in lighting/environment. But there is work to improve this.
- 3D Gaussian Ray Tracing: Fast Tracing of Particle Scenes tackles one part of this.
- StyleSplat: 3D Object Style Transfer with Gaussian Splatting; takes the approach of style-transfer to enable editing of 3D splats.
- RRM: Relightable assets using Radiance guided Material extraction.
- It is also hard to identify and modify objects in splat point-clouds.
- Let It Flow: Simultaneous Optimization of 3D Flow and Object Clustering; uses flow-based methods to cluster points.
- Click-Gaussian: Interactive Segmentation to Any 3D Gaussians; allows one to interactively pick objects, and then modify (move/rescale) them (video).
- Animate3D: Animating Any 3D Model with Multi-view Video Diffusion (video).
- Buildbox 4 has a platform for building games by iteratively prompting an AI.
Policy
- The US Department of Energy announced a new initiative: Frontiers in Artificial Intelligence for Science, Security and Technology (FASST). There is a senate bill proposing to fund this effort at $2.4B/year.
Education
- Andrej Karpathy has announced a new venture that will leverage AI to improve education. Eureka Labs will build AI teaching assistants to work alongside teachers in helping students understand complex topics. The company’s first concrete output is (naturally) a course focused on how to build an AI model (aimed at undergraduates).
Brain
- Scaling Law in Neural Data: Non-Invasive Speech Decoding with 175 Hours of EEG Data. They synthesize speech using EEG data fed through a neural model. They show that performance improves continually as a function of dataset size (up to 175 hours; by comparison usually people only use ~10 hours of data). The lack of plateau in the scaling is good news in the bitter lesson sense: it suggests that there is plenty of available performance by simply scaling up known methods on more and more brain data.
Consciousness
- A survey of the top 200 definitions of what consciousness might be: A landscape of consciousness: Toward a taxonomy of explanations and implications.
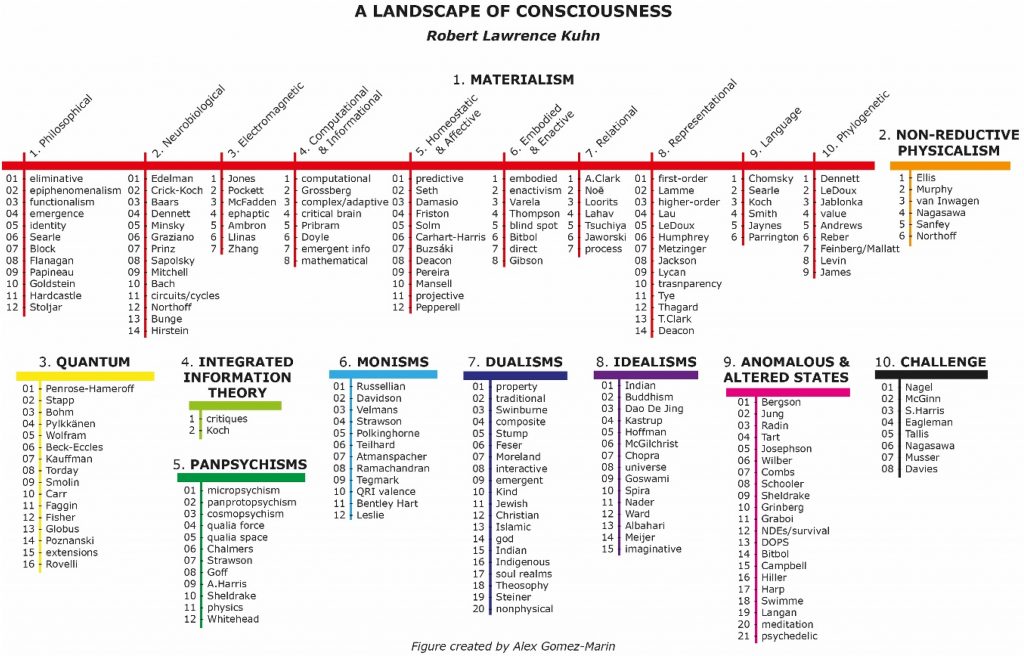
Hardware
Robots
- Disney published on the control approach for their cute bipedal robot: Design and Control of a Bipedal Robotic Character. Their reinforcement learning approach includes the usual (stability, locomotion) with artistic control aspects (including behaviors that humans find pleasing/amusing).
- DextrAH-G: Pixels-to-Action Dexterous Arm-Hand Grasping with Geometric Fabrics. Using reinforcement learning in simulation to train object-grasping policies. The transfer learning from sim to reality works remarkably well (videos).
- Robotic Control via Embodied Chain-of-Thought Reasoning. Chain-of-thought reasoning improves chatbot response. This approach has been applied to vision-language-action (VLA) models, improving task performance since it plans ahead (project page, videos).