General
- Noam Brown (OpenAI) spoke at TED AI on the importance of system 2 thinking for future AI. For poker, he notes how ~20 seconds of thinking gives the same boost as ~100,000× model scaling.
- There is growing evidence that some version of this also holds true for reasoning AI systems (based on LLMs), as seen in o1.
- According to the Verge: Google plans to announce its next Gemini model soon. They also repeat the rumor that OpenAI will release a new model (possibly in December 2024).
- Another report showing that uptake of genAI is strong: Growing Up: Navigating Generative AI’s Early Years – AI Adoption Report (executive summary, full report).
- 72% of leaders use genAI at least once a week (c.f. 23% in 2023); 90% agree AI enhances skills (c.f. 80% in 2023).
- Spending on genAI is up 130% (most companies plan to invest going forward).
- Sundar Pichai indicates that 25% of all new code at Google is generated by AI.
- News that OpenAI is seeking to develop its own chips for accelerated AI compute. This is being done in collaboration with Broadcom and TSMC.
- The US White House releases an AI memo, calling upon agencies to harness the power of AI.
Research Insights
- adi has proposed a new benchmark for evaluating agentic AI: MC bench (code). It consists of having the agent build an elaborate structure in Minecraft. By using humans to A/B rank the visual output, the capability of agents can be ranked.
- Anthropic have provided an update to their interpretability work, where the activation space is projected concisely into a higher-dimensional space using sparse auto-encoders (SAE). Now, they posted: Evaluating feature steering: A case study in mitigating social biases. Earlier work showed that they can enforce certain kinds of model behaviors or personalities by exploiting a discovered interpretable feature. Now, they further investigate; focusing on features related to social bias. They find that they can, indeed steer the model (e.g. elicit more neutral and unbiased responses). They also find that pushing too far away from a central “sweet spot” leads to reduced capabilities.
- RL, but don’t do anything I wouldn’t do. In traditional training, parts of the semantic space without data are simply interpolated. This can lead to unintended AI behaviors in those areas. In particular, this means when an AI isn’t sure what to do, they do exactly that undefined thing. This new approach tries to consider uncertainty. So when an AI isn’t sure about an action, it is biased towards not taking that action. This captures a sort of “don’t do anything I might not do” signals.
- Mixture of Parrots: Experts improve memorization more than reasoning. The “mixture-of-experts” method (of having different weights that get triggered depending on context) seems to improve memorization (more knowledge for a given inference-time parameter budget) but not reasoning. This makes sense; reasoning is more of an “iterative deliberation” process that benefits from single-pass parameters and multi-pass refinement.
- The Geometry of Concepts: Sparse Autoencoder Feature Structure. Tegmark et al. report on finding the feature space of LLMs spontaneously organizes in a hierarchical manner: “atomic” structures at small-scale, “brain” organization at intermediate-scale, and “galaxy” distribution at large-scale.
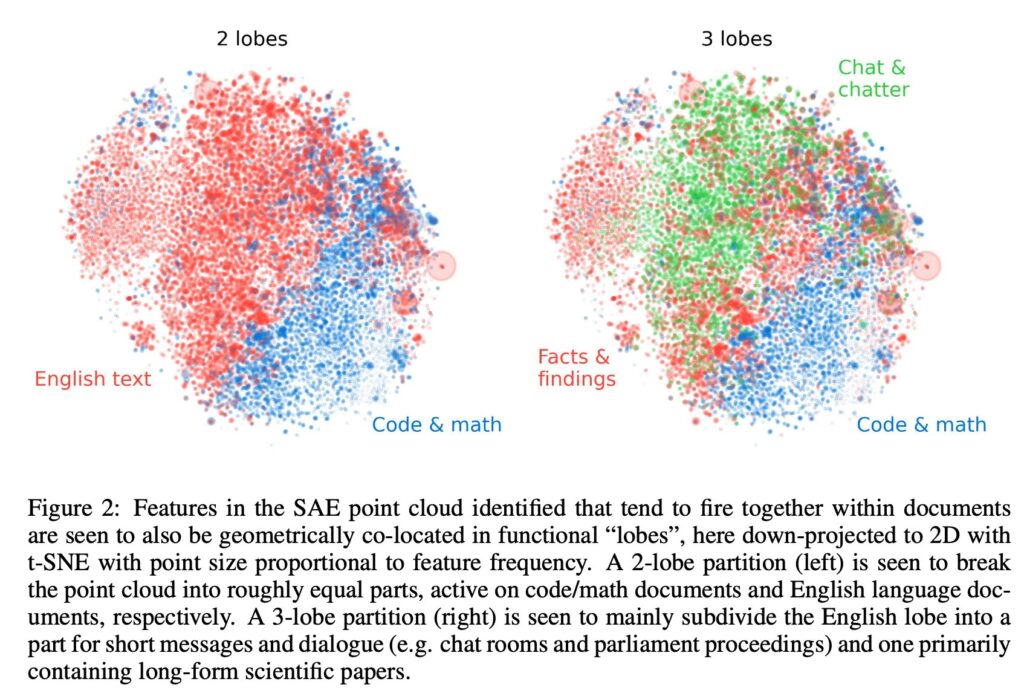
LLM
- Anthropic adds an “analysis tool” to Claude, allowing it to write and run JavaScript.
- Google’s Notebook LM “generate podcast” feature has spawned some open-source replication efforts: appeared: PDF2Audio (code), Open NotebookLM (code), and podcastfy (and ZenMic. product). Now, Meta released NotebookLlama, a recipe for building your own (example output).
- Microsoft describes: Data Formulator: Exploring how AI can help analysts create rich data visualizations (video, code).
- Github Spark is a new system for building apps, using natural language (promo video, demo video).
- Github Copilot now offers choice of more models (including Anthropic).
- Perplexity and Github Copilot announce an integration, allowing users to ask Perplexity questions from within their Copilot dev environment (through an extension).
- At OpenAI dev day, they announced some forthcoming o1 features: function calling, developer messages, streaming, structured outputs, image understanding. This would bring o1 up to the tools-capability of their other models.
- OpenAI open-sources SimpleQA (code), a benchmark for assessing factuality.
- OpenAI release their web search product to a broad range of users.
Audio
Image Synthesis
- Stable Diffusion released version 3.5 last week, and have now released the Stable Diffusion 3.5 Medium model.
- New image model: Recraft V3 (which was tested as “red panda” and was highly ranked by users).
Video
- MarDini: Masked Autoregressive Diffusion for Video Generation at Scale (project page with video examples). Seems to capture “physics” (fluids, gas, fire) quite well.
World Synthesis
- Autodesk’s Wonder Studio shows off the latest capabilities: mapping of live-action footage into a 3D environment, including world assets and characters with animations (example from beta tester). This gives the artist the best of both worlds: immediate generation of usable assets, with the ability to alter anything (environment, characters, movements, camera position) afterwards.
Robots
- Another video of the EngineAI SE01 robot walking around in a rather humanlike way.
- A video of Boston Dynamic’s Atlas robot (new, electric version) performing some autonomous tasks.
- Physical Intelligence shows off a simple two-armed robot autonomously performing a rather complicated task: laundry (and making coffee, picking up trash, etc.).