General
- Meta reveals that they are training Llama 4, and are using a cluster with >100k H100 GPUs.
- Miles Brundage offers another measured blog post: Should AI Progress Speed Up, Slow Down, or Stay the Same? I don’t know, and you don’t, either.
- Computerworld article: Agentic AI swarms are headed your way.
- Needless to say, I agree: Towards a Science Exocortex.
- Amazon’s new Alexa has reportedly slipped to 2025. It’s surprising, given Amazon’s lead (existing devices in homes, etc.) and considerable resources, that they have not been able to operationalize modern LLMs. Then again, I suppose the legacy capabilities and customer expectations (replacement must work at least as well, in myriad small tasks, as existing offering) slows down the ability to make changes.
- We might be seeing something similar play out with Apple’s promises of AI features.
- Google Claims World First As AI Finds 0-Day Security Vulnerability.
- New study on impacts of AI to workers: Artificial Intelligence, Scientific Discovery, and Product Innovation. They find that for R&D materials scientists, diffusion models increase productivity and “innovation” (patents), boost the best performers, but also remove some enjoyable tasks.
Research Insights
- Agent S: An Open Agentic Framework that Uses Computers Like a Human (code).
- Similar to Anthropic’s recently-announced computer use ability.
- TokenFormer: Rethinking Transformer Scaling with Tokenized Model Parameters. Treats model parameters as tokens, so that input queries become attentional lookups to retrieve model parameters. This leads to an efficiency improvement when scaling.
- How Far is Video Generation from World Model: A Physical Law Perspective (preprint, code, video abstract). They train a video model on simple physics interactions. The model generalizes perfectly within-distribution, but fails in general when extrapolating out-of-distribution. This implies the model is not learning the underlying physics.
- A valid question is whether they provided enough coverage in training, and enough scale (data, parameters, training compute) to actually infer generalized physics. It’s possible that at a sufficient scale, robust physics modeling appears as an emergent capability.
- Conversely, the implication might be that generalization tends to be interpolative, and the only reason LLMs (and humans?) appear generalized is that they have enough training data that they only ever need to generalize in-distribution.
- Mixtures of In-Context Learners. Allows one to extract more value from existing LLMs, including those being accessed via cloud (weights not available). The method creates a set of different “experts” by calling an LLM repeatedly with different in-context examples. Instead of just merging or voting on their final responses, one can try to consolidate their responses at the token level by looking at the distribution of predictions for next token. This allows one, for instance, to provide more examples than the context window allows.
- It would be interesting to combine this approach with entropy sampling methods (e.g. entropix) to further refine performance.
- AI swarms require communication between agents, but right now there are many competing methods for multi-agent coordination (Camel, Swarm, LangChain, AutoGen, MetaGPT). Researchers at Oxford have proposed a scheme (Agora) for AI agents can auto-negotiate a structured protocol: A Scalable Communication Protocol for Networks of Large Language Models (preprint).
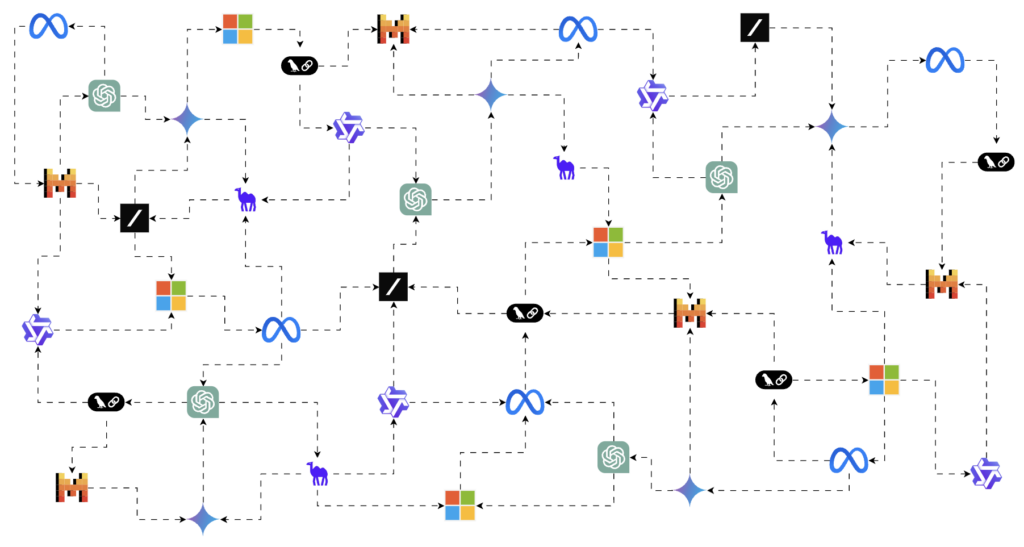
LLM
- Anthropic added visual PDF support to Claude. Now, when Claude ingests a PDF, it does not only consider a textual conversion of the document, but can also see the visual content of the PDF, allowing it to look at figures, layout, diagrams, etc.
- Anthropic releases Claude 3.5 Haiku, a small/efficient model that actually surpasses their older large model (Claude 3 Opus) on many benchmarks.
Tools
- Google is now making available Learn About, a sort of AI tutor that can help you learn about a topic. (Seems great for education.)
Image Synthesis
- The recently released Recraft V3 (highly ranked in blind tests under the “red panda” name) has the ability to generate SVG (e.g.).
- Black Forest Labs have released Flux 1.1 Pro Ultra, a high-resolution version of their image system. It can generate some extremely realistic images.
Audio
- Hertz-dev is an open-source audio foundation model, that can be adapted to various tasks.
Video
- Runway ML “advanced camera controls” are now available on the Gen-3 Alpha Turbo model.
- ByteDance unveils a superior lipsync model: X-Portrait 2: Highly Expressive Portrait Animation. Captures complex and dynamic facial performance (examples 1, 2, 3).
- Current quality of video generations:
World Synthesis
- Neural reproductions of video games are impressive. We’ve seen Doom, Super Mario Bros., and Counter-Strike.
- Now, Decart AI (working with Etched) are showing a playable neural-rendered video game (basically Minecraft). Playable here (500M parameters, code). Right now, this is just a proof-of-principle. There is no way for the game designer to design an experience, and the playing itself is not ideal (e.g. it lacks persistence for changes made to terrain). It feels more like a dream than a video game. But the direction this is evolving is clear: we could have a future class of video games (or, more broadly, simulation environments) that are designed using AI methods (prompting, iterating, etc.), and neural-rendered in real-time. This would completely bypass the traditional pipelines.
- To underscore why you should be thinking about this result in a “rate of progress” context (rather than what it currently is), compare: AI video 2022 to AI video today. So, think about where neural-world-rendering will be in ~2 years.
- And we now also have GameGen-X: a diffusion transformer for generating and controlling video game assets and environments.
- Now, Decart AI (working with Etched) are showing a playable neural-rendered video game (basically Minecraft). Playable here (500M parameters, code). Right now, this is just a proof-of-principle. There is no way for the game designer to design an experience, and the playing itself is not ideal (e.g. it lacks persistence for changes made to terrain). It feels more like a dream than a video game. But the direction this is evolving is clear: we could have a future class of video games (or, more broadly, simulation environments) that are designed using AI methods (prompting, iterating, etc.), and neural-rendered in real-time. This would completely bypass the traditional pipelines.
Science
- Anthropic’s “Golden Gate Claude” interpretability/control method consists of identifying legible features in activation space. Researchers have applied this mechanistic interpretability to understanding protein language models. They find expected features, such as one associated with the repeating sequence of an alpha helix or beta hairpin (visualizer, code, SAE). More fully understanding the learned representation may well give new insights into proteins.
- More generally, it is likely a very fruitful endeavor to train large models on science data, and search in a feature space for expected features (confirm it learned known physics), and thereafter search for novel physics in the space.
Robots
- Xpeng reveal their humanoid robot Iron (promo video, working in factory).