General
- Fei-Fei Li announced World Labs, which is: “a spatial intelligence company building Large World Models (LWMs) to perceive, generate, and interact with the 3D world”.
- Microsoft announces “Wave 2” of their Microsoft 365 Copilot (see also this video). Not much in terms of specifics, but the announcement reiterates the point (c.f. Aidan McLaughlin’s post) that as models become more powerful and commoditized, the “wrapper”/”scaffolding” becomes the locus of value. Presumably, this means Microsoft intends to offer progressively more sophisticated/integrated tools.
- Scale and CAIS are trying to put together an extremely challenging evaluation for LLMs; they are calling it “Humanity’s Last Exam”. They are looking for questions that would be challenging even for experts in a field, and which would be genuinely surprising if an LLM answered correctly. You can submit questions here. The purpose, of course, is to have a new eval/benchmark for testing progressively smarter LLMs. It is surprisingly hard to come up with ultra-difficult questions that have simple, easy-to-evaluate answers.
- Data Commons is a global aggregation of verified data. Useful to underpin LLM retrievals. It is being pushed by Google (e.g. DataGemma).
Research Insights
- IBM released a preprint: Automating Thought of Search: A Journey Towards Soundness and Completeness.
- This is based on: Thought of Search: Planning with Language Models Through The Lens of Efficiency (Apr 2024). This paper uses LLM for planning, emphasizing completeness and soundness of searching. Their design invokes the LLM less frequently, relying on more traditional methods to implement search algorithms. But, they use the LLM to generate the code required for the search (goal test, heuristic function, etc.). This provides some balance, leveraging the flexibility and generalization of the LLM, while still using efficient code-execution search methods.
- This new paper further automates this process. The LLM generates code for search components (e.g. unit tests), without the need of human oversight.
- Schrodinger’s Memory: Large Language Models. Considers how LLM memory works.
- LLMs + Persona-Plug = Personalized LLMs. Rather than personalize LLM response with in-context data (e.g. document retrieval), this method generates a set of personalized embeddings for a particular user’s historical context. This biases the model towards a particular set of desired outputs.
- More generally, one could imagine powerful base model, with various “tweaks” layered on top (modified embedding, LoRA, etc.) to adapt it to each person’s specific use-case.
Policy & Safety
- Sara Hooker (head of Cohere for AI) published: On the Limitations of Compute Thresholds as a Governance Strategy. Many proposed policies/laws for AI safety rely on using compute thresholds, with the assumption that progressively more powerful models will require exponentially more compute to train. The remarkable effectiveness/scaling of inference-time-compute partially calls this into question. The ability to distill into smaller and more efficient models is also illustrative. Overall, the paper argues that the correlation between compute and risk is not strong, and relying on compute thresholds is an insufficient safety strategy.
- Dan Hendrycks AI Safety textbook through CAIS.
LLM
- OpenAI announced o1, which is a “system 2” type methodology. Using reinforcement learning, they’ve trained a model that does extended chain-of-thought thinking, allowing it to self-correct, revise planning, and thereby handle much more complex problems. The o1 models show improvements on puzzles, math, science, and other tasks that require planning.
- It was initially rate-limited in the chat interface to 50 messages/week for o1-mini, and 30 messages/week for o1-preview. This was then increased to 50 messages/day (7× increase) and 50 messages/week (~1.7×).
- It has rapidly risen to the top of the LiveBench AI leaderboard (a challenging LLM benchmark).
- Ethan Mollick has been using an advanced preview of o1. He is impressed, noting that in a “Co-Intelligence” sense (human and AI working together), the AI can now handle a greater range of tasks.
- The OpenAI safety analysis shows some interesting behavior. The improved reasoning behavior also translates into improved plans for circumventing rules or exploiting loopholes, and provides some real-world proof of AI instrumental convergence towards power-seeking.
- In an AMA, the o1 developers answered some questions; summary notes here.
- Artificial Analysis provides an assessment: “OpenAI’s o1 models push the intelligence frontier but might not make sense for most production use-cases”.
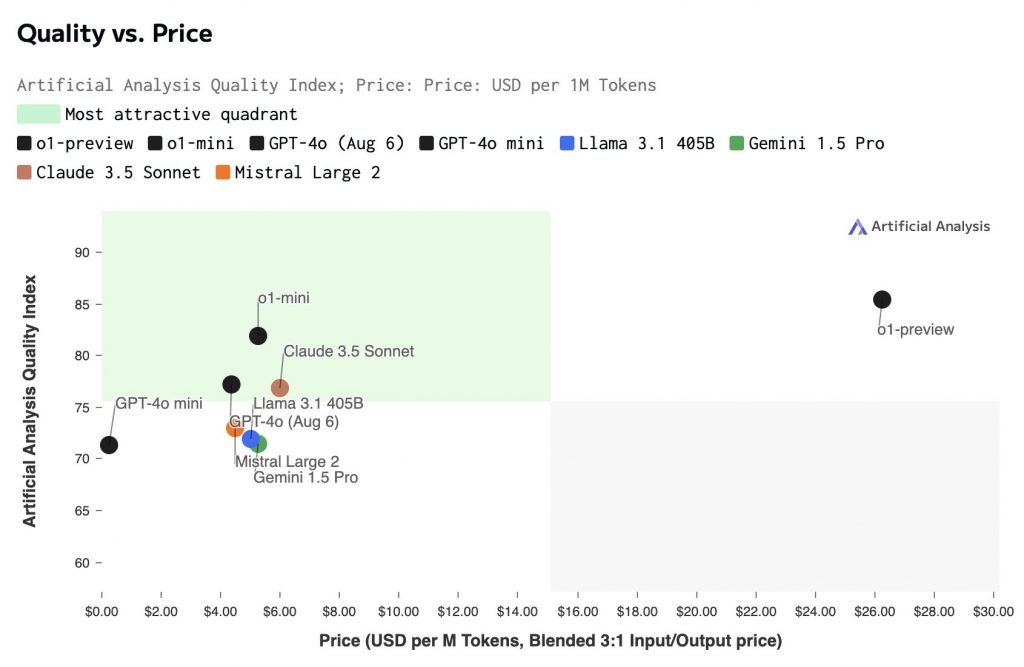
- Kyutai have open-sourced their Moshi voice chatbot (video, online demo): model weights, inference code.
- They also released a technical report: Moshi: a speech-text foundation model for real-time dialogue.
- NVLM 1.0 claims strong performance on language/vision tasks; they say the weights/code will be released soon.
- Microsoft released GRadient-INformed (GRIN) MoE 6.6B model (demo, models, github). From the metrics they report, this puts their model in a remarkable place in terms of performance/parameter.
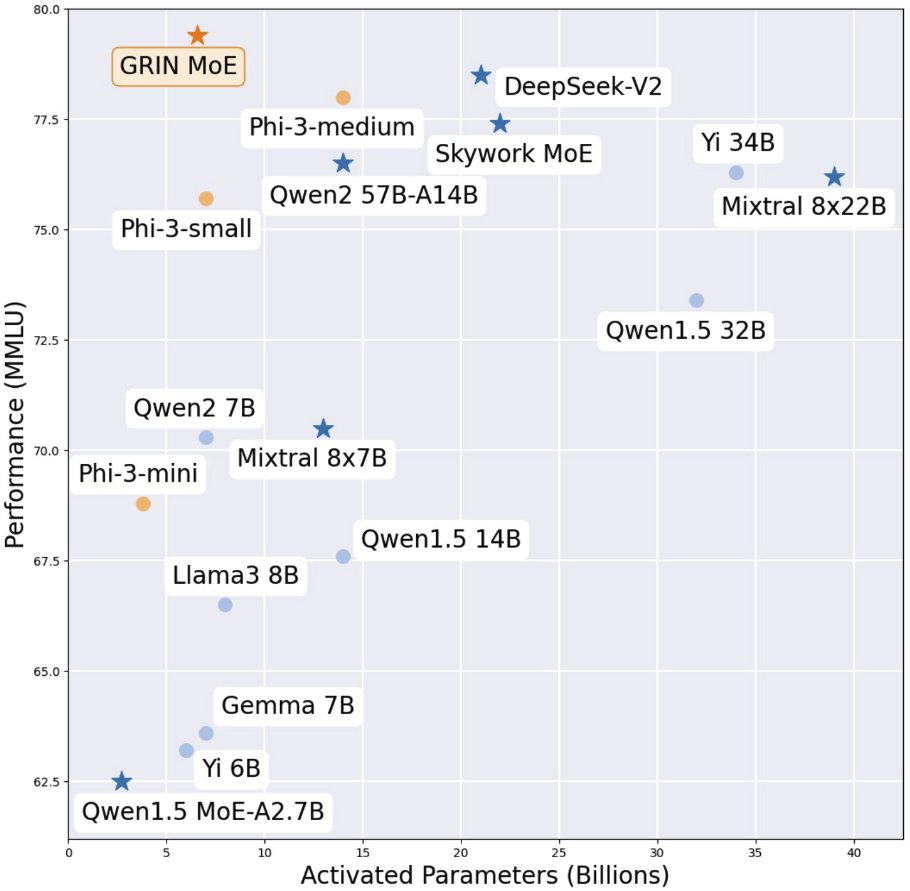
- An open-source competitor for LLM-enhanced code editors (like Cursor): Void code editor (github).
- Slack have announced a pricier tier: Slack AI. It will be an “AI agent hub” where AIs join conversations to summarize and otherwise contribute.
Voice
- Google’s Notebook LM has added a “Generate” button that will create a “podcast” discussion of the provided sources, where two people back-and-forth discuss. (This was previously developed as the Illuminate experiment.) It’s a simple trick, but remarkably effective at creating something that feels engaging (example 1, example 2).
Vision
- SAM 2 Studio is a fully local app for image segmentation (models, github, medical segmentation example). Obviously based on Segment Anything Model 2.
- World-Grounded Human Motion Recovery via Gravity-View Coordinates (paper, code). Yields pose/motion estimation from a single-camera (monocular) video (no need for tracking dots/suits).
Image Synthesis
- Drag-based image editing seems like a good UI for generative image editing.
Video
- HuggingFace released an open dataset for video: FineVideo. This should help open-source genVideo efforts catch up. (Currently all the best video generators are closed.)
- PoseTalk is another approach for animating a single photo to match a provided audio.
- HeyGen released Avatar 3.0; the animated AI avatars are improved (greater body motion, dynamic voice tones, etc.).
- Facecam AI is another real-time face swapping system (seems to be based on Deep-Live-Cam).
- Google has released an AI-powered video creation app. It is showing up in Google Drive for some users. This is focused on “video powerpoint” style presentations suitable for corporate settings or perhaps YouTube videos.
- Runway ML:
- Added a video-to-video capability to their Gen 3 system. You can prompt to restyle or otherwise transform the input video. (Examples here; including some where the restyled video is comped back onto the original footage, using the genAI as a VFX.)
- Announced a partnership with Lionsgate.
- Added a developer API, allowing programmatic generation of videos (using the turbo model).
- Luma Labs have also added an API for Dream Machine.
- Kling released a 1.5 update to their video model (example outputs, showing emotions).
- New genAI video model: Vchitect-2.0 (demo, model, code, examples).
- Snap has announced a forthcoming video model, for creators inside Snapchat.
- Current batch of genAI video examples:
- Runway video-to-video to restyle classic video games; not hard to imagine a real-time version of this being used in future video games
- Realistic presenter
- Skateboarding (demonstrates getting closer to meaningfully simulating motion/physics)
- Examples of short clips with cinematic feel
- Short: 4 Minutes to Live.
- Short: Neon Nights (Arcade).
- Random Access Memories. AI-generated, but then projected onto Kodak film stock. Gives the final output some of the dreamy analog quality we associate with nostalgic footage.
World Synthesis
- Gaussian Garments: Reconstructing Simulation-Ready Clothing with Photorealistic Appearance from Multi-View Video (preprint).
- Application of 3D Gaussian Splatting for Cinematic Anatomy on Consumer Class Devices (preprint, code). Some amazing-looking movies.
- FlashSplat: 2D to 3D Gaussian Splatting Segmentation Solved Optimally (code). Enables correct segmentation of 3D objects in Gaussian splat scenes, using just 2D segmentation/masking.
- Dynamic Gaussian Marbles for Novel View Synthesis of Casual Monocular Videos (preprint, code). Enables conversion from video (3D) into dynamic environment (4D).
- GameGen-O: Open-world Video Game Generation (github). Diffusion-transformer generation of game environments, characters, and actions/events. This doesn’t seem to output a fully playable game, but does seem to be generating many of the required components.
Hardware
- Snap’s 5th-generation Spectacles are AR glasses. These are intended for developers. Specs are: standalone, 46° FOV, 37 pixels per degree (~100” screen), 2 snapdragon chips, 45 minutes of battery, auto transitioning lenses.
Robots