Research Insights
Several results relevant to recursive self-improvement:
- LLMs are trained on human text, of which there is a finite amount. Some estimates put the ‘data wall’ (running out of larger training datasets on which to train ever-larger models) in 2027-2028. A possible solution is to use AI to generate synthetic training data. Is that a good idea?
- New paper: AI models collapse when trained on recursively generated data. Adds to the body of work that shows that repeatedly training new models on synthetic data generated by previous models reduces diversity and eventually causes the model to collapse into garbage.
- Also studied previously for images (stability, MAD) and text (models forget, knowledge collapse).
- However, these results have been criticized as being unrealistic of how synthetic data training occurs in practice. Prior studies have tended to progressively replace all original data with synthetic. In practice, synthetic data is used to augment the original training set. Thus data accumulation, focused generation, and reinforcement can avoid model collapse.
- LLM training on synthetic data is not just theoretical. The recently-release Llama 3.1 used a variety of synthetic data augmentation methods.
- LLMs are notoriously bad at math. There are many approaches to fix this, including giving the LLM access to tools (calculator, Python) or using special encodings for numbers. However, with the right training scheme even a GPT-2 class model can learn to multiply numbers.
- Preprint: From Explicit CoT to Implicit CoT: Learning to Internalize CoT Step by Step. They start with a model that does explicit chain-of-thought to come to the right answer, and then progressively remove intermediate steps so that the underlying logic becomes internalized in the model. They show it works for 20 digit numbers (demo).
- Distillation (e.g. training a small model on the output of a bigger one) broadly also shows that complex thoughts can be compactly internalized. This bodes well for model self-play, where it searches problem-spaces in a computationally expensive manner, but progressively internalizes the corresponding complexity.
- Preprint: Recursive Introspection: Teaching Language Model Agents How to Self-Improve. The LLM detects and corrects its own mistakes, which is used to iteratively fine-tune the model in an RL scheme.
- Preprint: Self-Discover: Large Language Models Self-Compose Reasoning Structures. LLM selects and combines reasoning structures to solve tasks.
- Preprint: Meta-Rewarding Language Models: Self-Improving Alignment with LLM-as-a-Meta-Judge. LLMs can self-improve by generating outputs and judging the quality of their own outputs. However, improvements tend to saturate. This new work uses a meta approach where the LLM also judges its judgements, in order to progressively improve its own judging. This expands the range of possible improvement; while still being fully unsupervised.
Some new work investigates LLM reasoning and compute tradeoffs:
- The Larger the Better? Improved LLM Code-Generation via Budget Reallocation. Larger models are better. But for a tested coding task, a smaller budget given more execution time could outperform a larger model given the same compute budget. This is surprising in the sense that a sufficiently small model will presumably under-perform, no matter how much compute it is given. But across a range of meaningful tasks, smaller models can yield more compute-efficient results.
- Large Language Monkeys: Scaling Inference Compute with Repeated Sampling. Another approach that involves inference-time compute (“search”) to improve smarts. It also shows that repeated calls to simple models can out-perform a larger model. The method is strong successful where a verifier is available; much harder when those are lacking.
- Physics of Language Models: Part 2.1, Grade-School Math and the Hidden Reasoning Process.
- Models learn reasoning skills (they are not merely memorizing solution templates). They can mentally generate simple short plans (like humans).
- When presented facts, models develop internal understanding of what parameters (recursively) depend on each other. This occurs even before an explicit question is asked (i.e. before the task is defined). This appears to be different from human reasoning.
- Model depth matters for reasoning. This cannot be mitigated by chain-of-thought prompting (which allow models to develop and then execute plans) since even a single CoT step may require deep, multi-step reasoning/planning.
There are conflicting messages here. You can trade-off between model complexity/power and repeated calls to that model. Is it better to use a large/smart model, or better to repeatedly call a small model? For some problems, iterating or searching using a small model is better. But there are cases where individual steps are sufficiently complex that they require properly parallel/coherent attention among disparate elements. So in that case you need the power of the large model. This still points in a familiar direction: models should be made more powerful (so that system-1 intuition becomes as refined as possible), but should also be wrapped in a way that lets them iterate on tasks (so that more explicit system-2 iteration/search can occur).
Other research results:
- Knowledge Overshadowing Causes Amalgamated Hallucination in Large Language Models. They identify that non-truth-grounded hallucinations may arise from imbalance in training data, such that the LLM over-generalizes. So they can detect and mitigate hallucinations.
- Other works have shown that hallucinations are in some sense beneficial, for creativity and even reasoning (for narrative and communication). So it would be interesting to know whether this mitigation decreases creativity.
Safety
- NIST released draft AI safety guidelines: Managing Misuse Risk for Dual-Use Foundation Models. Now open for public comments.
Policy
- OpenAI posted their interpretation of the EU AI Act.
- Microsoft releases report: Generative AI in Real-World Workplaces. There is growing evidence of genAI improving productivity, though the gains realized so far are generally modest and vary substantially by job type and work context.
- Manhattan Institute posts A Playbook for AI Policy. (Contains similar arguments to Leopold Aschenbrenner‘s Situational Awareness, c.f.) Argues that the US must maintain strategic lead in AI.
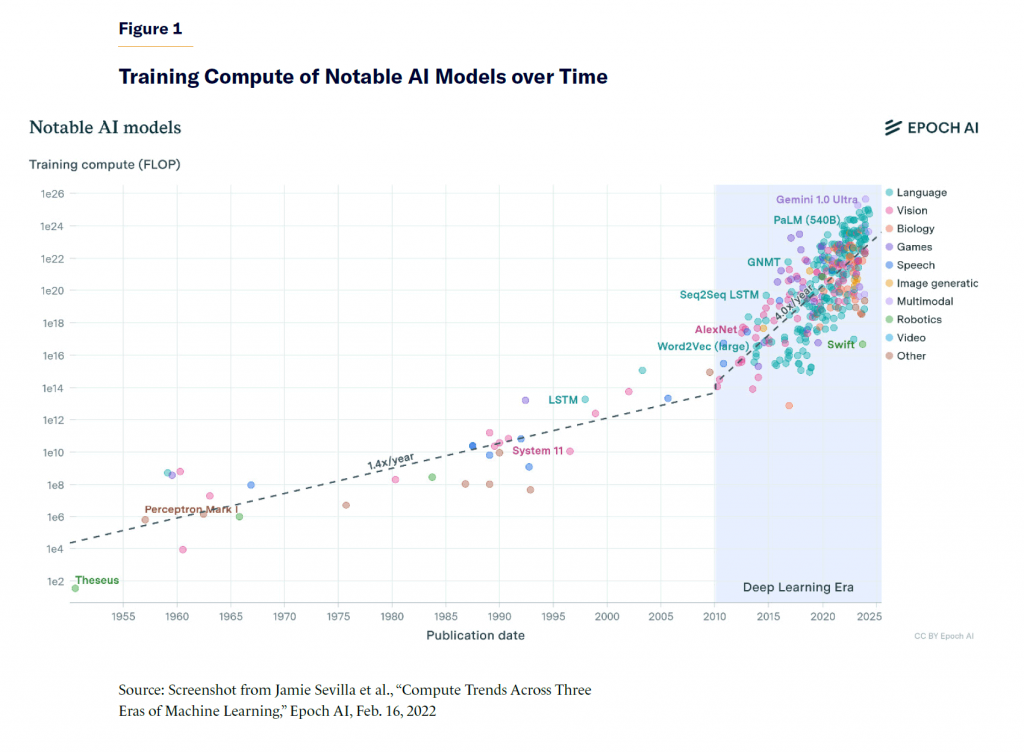
LLMs
- Anthropic is reportedly working on a folder-sync feature, to streamline interacting with the LLM on projects.
- OpenAI is having some users alpha-test GPT-4o with a long output (64k tokens).
- Topology AI opened a demo of their chatbot. The novelty of their offering is a Continuous Learning Model (CLM), which they claim “remembers interactions, learns skills autonomously, and thinks in its free time”. The documents describe this as being distinct from fine-tuning or document retrieval, and note using a combination of open-source and proprietary LLMs. It sounds vaguely like RAG on past conversations, but inserted more directly into the model than simply copy-pasting into the context window. Conceptually, model memory is definitely something future chatbots need.
- Google a Gemma 2 2B model. In the small-model regime, it seems to be doing quite well. It is small enough that it can run on smartphones, and is open-weights.
- Google made an experimental version (0801) of Gemini 1.5 Pro available (Google AI Studio). There are no details about what makes this model different. The LMSYS leaderboard currently puts it at the #1 spot overall. Some are disputing the rank and worrying that the benchmarks are not correctly capturing reasoning power. Nevertheless, seems like an impressive achievement from Google.
- SambaNova has a demo of running LLMs extremely fast (using their custom hardware).
- Some folks formulate the baba-is-ai benchmark (preprint), where the AI must play the Baba Is You puzzle video game, which involves manipulating your character, the environment, and the game rules themselves. AIs currently fail horribly at this task, which makes it a good test-bed for improved reasoning.
Image Synthesis
- Midjourney released v6.1 which features improved image quality and coherence.
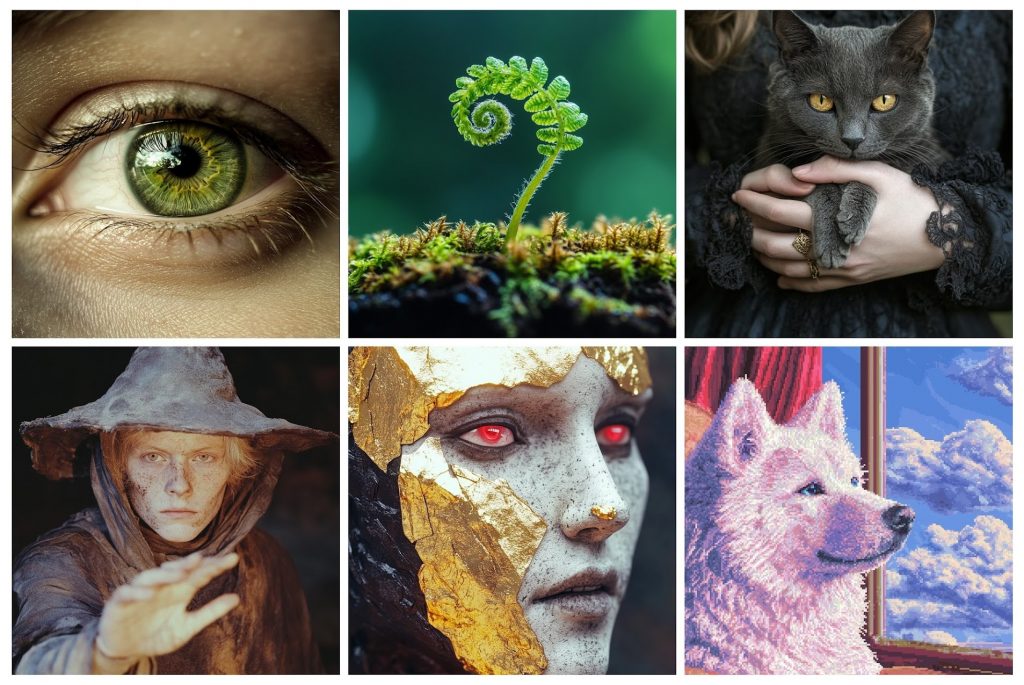
Vision
- Meta released Segment Anything Model 2 (SAM2); a follow-up to their very successful SAM. SAM2 can segment/isolate objects in images, and in video data (with excellent temporal consistency). Apparently fast enough for real-time and interactive use (demo). Can handle very complex multi-object scenes. Interestingly, it can even track objects/people across cuts and scene changes. Applications in video editing software (compositing, etc.) are obvious. But it should also be relevant for robotic vision or other automated video analysis. A quick test shows that it could also do segmentation in 3D medical imaging contexts.
- Combining with T-Rex2 allows tracking multiple objects with a single prompt.
- TAPTR (Tracking Any Point with TRansformer) does robust point-tracking in video data (examples, point demo, area demo).
Video
- Clapper is an open-source in-browser video editor that uses AI.
- HumanVid allows video generation with control of camera and character-pose.
- Runway ML Gen-3alpha text-to-video system:
- Added image-to-video
- Announced a turbo version; 7× faster (and cheaper)
- Generative video and music keep improving. Here’s a nice example of what can now be accomplished.
World Synthesis
- HoloDreamer: Holistic 3D Panoramic World Generation from Text Descriptions. We are getting closer to the goal of generative virtual worlds.
- Nvidia introduces fVDB, a deep learning framework to facilitate large/complex world representations (for autonomous driving, climate, etc.).
- GaussMR adds interactive particles and fluids to Gaussian splat scenes.
Hardware
- There is continued interest in making an AI Companion hardware device of sorts. The Humane AI Pin ($700) and Rabbit R1 ($200) did not receive strong reviews; mostly since they over-promised and under-delivered with respect to the AI capabilities. A new wave of options appear to be making more modest claims:
- The Limitless clip-on ($100) can record meetings, conversations, or spoken-aloud thoughts. It can then do straightforward transcription and AI summarization.
- Compass necklace ($100) similarly records and transcribes.
- Crush ($130) is a simple pushbutton voice recorder with AI summaries.
- Friend ($100) is a necklace that listens to your life, and the AI companion periodically messages you thoughts. You can also press a button to explicitly talk to it. This seems to be targeting wellness and fun (not utility). The advertising video left many wondering if this is satire. While there will undoubtedly be downsides and social changes associated with AI companions, one recent study shows that AIs can indeed alleviate loneliness.
- Confusingly, there is another AI-companion-via-pendant also called Friend (wearable necklace, $70); more focused on utility (transcription, summarization). The two Friend startups are not friendly with one another.
Robots
- Nvidia updated their Project GR00T robotic effort. They take video of humans performing tasks, and do data augmentation in a simulation environment (RoboCasa) with generative actions (MimicGen).
- Unitree robot-dog Go2 just got upgraded with wheels. This affords it the flexibility of walking over rough terrain but driving in flat areas. The previous Go2 was priced at $1,600.